What do they have in common?
When Dr. Christine Ford's accusation against now Justice Kavanaugh arrived with all the subtlety of the stricken Hindenburg, there was one thing that near as dammit to certain: the correlation between political proclivity and assault assessment.
Which is, or should be, beyond odd.
After all, in as much as they occupy entirely different realms, judicial philosophy and inclination towards coerced sex don't have any obvious correlation.
Yet when Dr. Ford's accusation came to light, the correlation between attitude towards constitutional originalism and Dr. Ford's credibility was nearly one. Progressives almost without exception found Dr. Ford credible; conservatives, incredible.
Same goes for Anthropogenic Global Warming. Conservative ≅ disdainful. Progressive ≅ dainful. Yet AGW, as an objective fact, just as Dr. Ford's accusation, is completely independent of judicial philosophy or political priors. These strong relationships shouldn't exist, yet there they are, nonetheless.
Welcome to motivated reasoning.
Clearly, a great many people simply do not think things through independently of their desire for a preferred outcome. Kavanaugh is to be resisted, therefore any impeachment of his character is true, and to heck with that bothersome evidence nonsense.
And just as clearly, should one have settled on individualistic free markets as the sine qua non of human flourishing, then AGW cannot, must not, be true.
Of course, as should be transparently obvious to even the most casual observer of reality, I am uniquely immune to motivated reasoning.
No matter that I agree with constitutional originalism, I am certain that Dr. Ford is a moral cretin.
And completely disregard the fact I am an individualist, AGW is nothing more than scientistic catechisms.
My reasoning is entirely unmotivated.
Now you know.
Forum for discussion and essays on a wide range of subjects including technology, politics, economics, philosophy, and partying.
Search This Blog
Thursday, February 14, 2019
Tuesday, February 05, 2019
Deep Learning and Emergent Deception
With all of the processing power available, all kinds of Deep Neural Network learning topologies are possible with tens of millions of connections or "parameters" (which are similar in purpose to synapses in a biological brain).
One of the more interesting nets to me are Generative Adversarial Networks (GANs) which are two (or more) connected networks that fight to win in a game to "outsmart" the other network. I've written about synthetic face generation before, and those applications use GANs. One network in the GAN learns to distinguish between real faces and synthetic faces and is called the discriminative network. The other network learns to generate synthetic faces and, not surprisingly, is called the generative network. The generative network is "rewarded" when a synthetic face is so realistic that it fools the discriminative network and "punished" when the discriminative network correctly identifies that the face is synthetic and not real. And when the generative network is rewarded, the discriminative network is punished and vice-versa. The two networks are locked in this zero sum win at all costs struggle, each trying to be rewarded and avoid punishment. If the GAN is set up correctly (being correct is mostly guesswork and trial and error), it can provide really impressive results as with the case of the synthetic faces.
But deception is an inherent part of the generative network. After all, it's designed to try an fool the discriminative network and ultimately us humans. Recently, a generative network went well past the bounds of deception expected by its creators. The application is this: transform aerial images into street maps and back to automate much of the image processing for things like google maps.
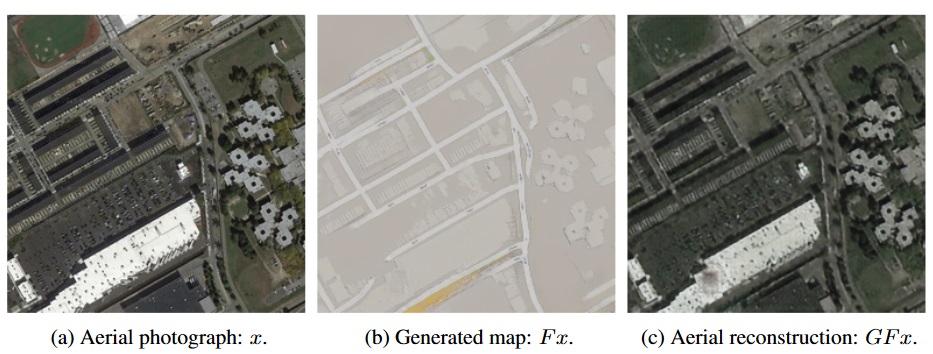
The above images show the process. There's the original aerial photograph (a), the street view (b), and the synthetic aerial view (c) that's reconstructed ONLY from the street view (b).
But wait! Looking at image (c), which is constructed from ONLY image (b), how on earth did it guess where to put the air conditioning units on the long white build? Or the trees? None of those details are in the street view image (b), right?
It turns out that the network "decided" to cheat:
The thing I find most interesting is the emergent deception. Nobody predicted this would happen (since it wasn't a desired result) and I don't think anybody could've predicted it.
We're currently able to use multiple networks with hundreds of millions of connections and we're already seeing emergent behavior that can't be predicted. Every ten years gives about a factor of 100 increase in processing power and network complexity.
It will be interesting to see what emerges when thousands of networks with billions of connections interact.
One of the more interesting nets to me are Generative Adversarial Networks (GANs) which are two (or more) connected networks that fight to win in a game to "outsmart" the other network. I've written about synthetic face generation before, and those applications use GANs. One network in the GAN learns to distinguish between real faces and synthetic faces and is called the discriminative network. The other network learns to generate synthetic faces and, not surprisingly, is called the generative network. The generative network is "rewarded" when a synthetic face is so realistic that it fools the discriminative network and "punished" when the discriminative network correctly identifies that the face is synthetic and not real. And when the generative network is rewarded, the discriminative network is punished and vice-versa. The two networks are locked in this zero sum win at all costs struggle, each trying to be rewarded and avoid punishment. If the GAN is set up correctly (being correct is mostly guesswork and trial and error), it can provide really impressive results as with the case of the synthetic faces.
But deception is an inherent part of the generative network. After all, it's designed to try an fool the discriminative network and ultimately us humans. Recently, a generative network went well past the bounds of deception expected by its creators. The application is this: transform aerial images into street maps and back to automate much of the image processing for things like google maps.
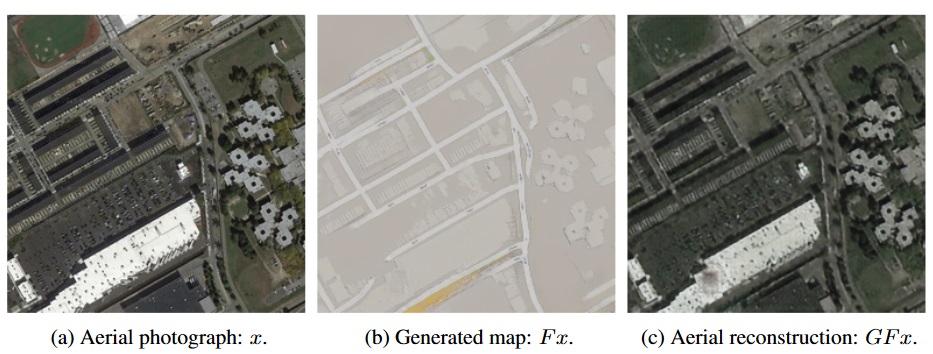
The above images show the process. There's the original aerial photograph (a), the street view (b), and the synthetic aerial view (c) that's reconstructed ONLY from the street view (b).
But wait! Looking at image (c), which is constructed from ONLY image (b), how on earth did it guess where to put the air conditioning units on the long white build? Or the trees? None of those details are in the street view image (b), right?
It turns out that the network "decided" to cheat:
It learned how to subtly encode the features of one into the noise patterns of the other. The details of the aerial map are secretly written into the actual visual data of the street map: thousands of tiny changes in color that the human eye wouldn’t notice, but that the computer can easily detect.In other words, the street view map has gazillions of minute variations that aren't visible to the human eye that encode the data required for the remarkable aerial reconstructions.
In fact, the computer is so good at slipping these details into the street maps that it had learned to encode any aerial map into any street map! It doesn’t even have to pay attention to the “real” street map — all the data needed for reconstructing the aerial photo can be superimposed harmlessly on a completely different street map...
This practice of encoding data into images isn’t new; it’s an established science called steganography, and it’s used all the time to, say, watermark images or add metadata like camera settings. But a computer creating its own steganographic method to evade having to actually learn to perform the task at hand is rather new.Note the last sentence. The generative network wasn't very good at generating the reconstructed aerial view the way it was supposed to. So instead, it figured out how to encode the data it needed so it didn't have to learn how to do it the right way.
The thing I find most interesting is the emergent deception. Nobody predicted this would happen (since it wasn't a desired result) and I don't think anybody could've predicted it.
We're currently able to use multiple networks with hundreds of millions of connections and we're already seeing emergent behavior that can't be predicted. Every ten years gives about a factor of 100 increase in processing power and network complexity.
It will be interesting to see what emerges when thousands of networks with billions of connections interact.
Subscribe to:
Posts (Atom)